Electric Vehicle Dataset
Discover comprehensive Electric Vehicle Datasets tailored for researchers, developers, and analysts. Our datasets provide insights into EV performance, charging infrastructure, battery capacities, range statistics, and more. Perfect for powering machine learning models, studying market trends, or supporting data-driven decisions in EV development and sustainability research.
Explore a vast range of data points including geographical, environmental, and technical specifications, curated to enhance understanding of electric vehicle technology and adoption worldwide. Whether for academic, business, or governmental use, our Electric Vehicle Dataset offers valuable, high-quality data to drive innovations in the evolving EV ecosystem.
Table of Contents
Electric Vehicle Dataset: An In-Depth Analysis for Modern Insights
The emergence of electric vehicles (EVs) is reshaping the global automotive industry, addressing climate change, reducing urban air pollution, and paving the way for sustainable transportation. Accurate and comprehensive datasets are crucial for understanding EV trends, consumer behavior, charging infrastructure, and policy impacts. In this post, we’ll explore the various dimensions of Electric Vehicle datasets, their significance, and how they are utilized.
What Is an Electric Vehicle Dataset?
An Electric Vehicle (EV) dataset comprises a collection of data points related to electric vehicles. These datasets can cover various aspects, including vehicle performance, sales statistics, charging behavior, battery longevity, environmental impact, and more. The information contained can be sourced from government databases, automobile manufacturers, private research firms, and real-world studies conducted on EV adoption.

Types of Electric Vehicle Datasets
Understanding the different types of EV datasets is crucial. Let’s break them down:
1. EV Sales and Market Statistics
- Description: This dataset tracks the sales figures of electric vehicles globally, regionally, or by country. It includes data on new registrations, market share comparisons, and growth trends over time.
- Key Variables: Year, Make and Model, Country, Units Sold, Percentage of Market Share, Growth Rate.
- Usage: Helpful for market analysts, investors, and automobile companies in tracking the growth and popularity of EVs.
2. Charging Infrastructure Data
- Description: Details on EV charging stations, including their location, availability, type (Level 1, Level 2, or DC fast charging), and operational status.
- Key Variables: Location (Latitude and Longitude), Type of Charger, Number of Ports, Charging Speed (kW), Accessibility (Public or Private), Provider.
- Usage: Used by city planners, businesses, and governments to optimize and expand the charging network.
3. Battery Performance and Longevity
- Description: Information on the performance of different EV batteries over time, including degradation rates, charging cycles, and range under various conditions.
- Key Variables: Battery Type (Lithium-ion, Solid-state), Capacity (kWh), Degradation Rate, Temperature Impact, Range per Charge, Charging Time.
- Usage: Valuable for engineers, battery manufacturers, and researchers working to enhance battery technology and EV efficiency.
4. Environmental Impact Data
- Description: Tracks the environmental benefits of using electric vehicles compared to traditional internal combustion engine vehicles, focusing on reduced emissions and energy efficiency.
- Key Variables: CO2 Emissions Saved (per km), Energy Consumption (kWh/100 km), Source of Electricity, Lifecycle Analysis (Manufacturing to Disposal).
- Usage: Used by environmentalists, researchers, and policymakers to quantify the benefits of EV adoption.
5. Consumer Behavior and Preferences
- Description: Insights into what motivates consumers to buy EVs, their concerns, and driving patterns.
- Key Variables: Demographics, Reasons for Purchase (Environmental, Cost Savings, Innovation), Barriers to Adoption (Range Anxiety, Cost), Average Daily Mileage.
- Usage: Helps auto manufacturers and marketers tailor their products and campaigns to consumer needs.
Popular Sources of Electric Vehicle Datasets
Access to accurate and high-quality data is essential for conducting meaningful analysis. Here are some key sources for EV datasets:
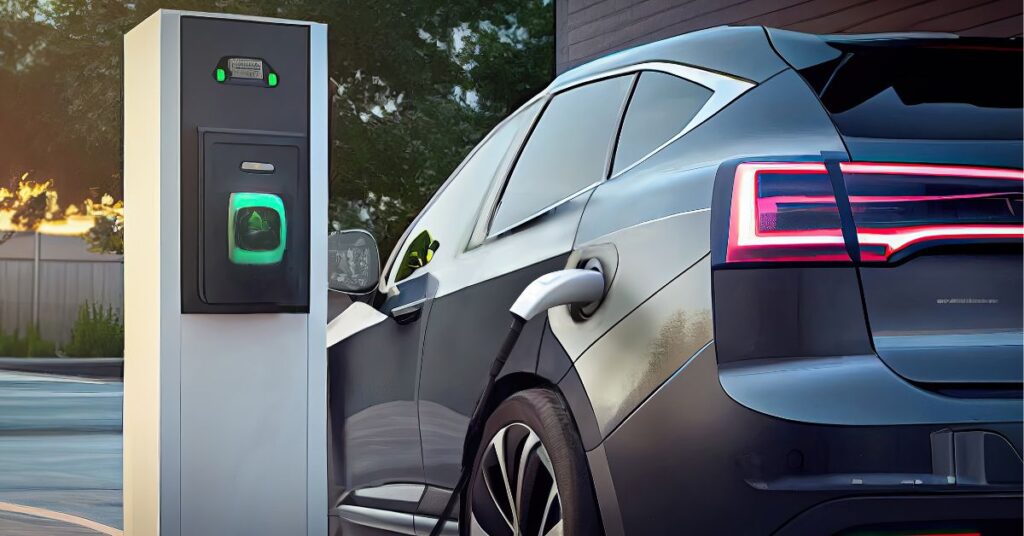
1. Government and Regulatory Bodies
- United States Department of Energy (DOE): The DOE provides comprehensive data on EV sales, charging stations, and research on energy consumption.
- European Alternative Fuels Observatory (EAFO): Offers data on the adoption of alternative fuels, including electric vehicles, across Europe.
- China Association of Automobile Manufacturers (CAAM): An authoritative source for EV market data in China, the world’s largest EV market.
2. Automobile Manufacturers
- Companies like Tesla, Nissan, and BMW often release reports and datasets on the performance of their electric models, sales figures, and customer satisfaction surveys.
3. Private Research Firms and Industry Reports
- BloombergNEF: Provides projections and market analysis on EV trends.
- Navigant Research: Specializes in tracking technological advancements and consumer behavior related to electric vehicles.
4. Open Data Platforms
- Kaggle: An online community of data scientists that offers numerous EV-related datasets for data analysis projects.
- OpenStreetMap: Contains data on charging station locations worldwide, which can be integrated with geospatial analysis tools.
5. Academic and Research Institutions
- Universities and think tanks publish studies and datasets on battery performance, urban mobility, and the environmental impact of EVs.
How to Use Electric Vehicle Datasets for Analysis
Analyzing EV datasets requires a thoughtful approach. Here are some common methods and tools:
1. Statistical Analysis and Data Visualization
- Tools: Python (pandas, matplotlib, seaborn), R, Tableau.
- Purpose: To identify trends, outliers, and correlations within datasets. For instance, one might analyze sales trends over a decade to forecast future market dynamics.
2. Geospatial Analysis
- Tools: ArcGIS, QGIS, Google Earth Engine.
- Purpose: Mapping charging station locations and analyzing accessibility across urban and rural areas. This helps optimize the placement of future charging points.
3. Machine Learning Models
- Use Case: Predicting battery degradation or sales trends using historical data.
- Algorithms: Linear regression for trend analysis, clustering for consumer segmentation, or time series forecasting models like ARIMA.
4. Lifecycle Analysis (LCA)
- Purpose: To evaluate the total environmental impact of an EV, from production to disposal. This can help determine the sustainability of different EV models compared to traditional vehicles.
Challenges in Electric Vehicle Data Analysis
While EV datasets are incredibly informative, several challenges need to be addressed:
1. Data Quality and Availability
- Not all data is consistently available across different regions. For example, while the U.S. and Europe have extensive datasets, data from developing countries may be sparse.
- Missing or incomplete data can lead to inaccurate analysis.
2. Data Privacy Concerns
- Consumer behavior datasets often contain sensitive information that must be anonymized to protect privacy.
- Regulations like the General Data Protection Regulation (GDPR) impose strict requirements on data usage.
3. Rapidly Evolving Technology
- The fast-paced advancements in EV technology mean that data can become outdated quickly. For instance, battery performance data from a decade ago may not reflect current capabilities.
4. Integration with Other Data Sources
- Combining EV datasets with other data, such as traffic patterns or renewable energy production, can be complex but is often necessary for comprehensive analysis.
Case Studies Using Electric Vehicle Datasets
Let’s look at some real-world examples where EV datasets have been pivotal:
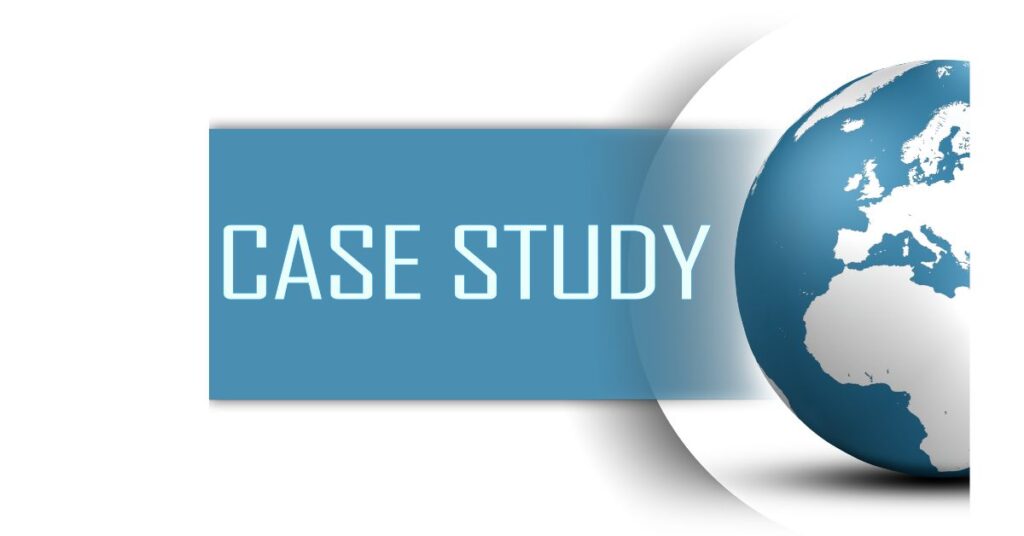
1. Optimizing Charging Infrastructure in Cities
- Problem: Cities need to ensure that EV drivers have access to convenient charging stations without oversaturating certain areas.
- Solution: Geospatial analysis of charging station usage patterns, combined with demographic data, helps city planners decide where to install new chargers.
- Impact: Improved accessibility for EV users and better utilization of public resources.
2. Predicting Battery Degradation
- Research: Several studies have used machine learning models to predict how quickly EV batteries degrade based on factors like temperature, charging habits, and mileage.
- Outcome: These predictions allow manufacturers to offer better warranties and help consumers make informed decisions about battery maintenance.
3. Assessing Environmental Benefits
- Analysis: Lifecycle analysis using environmental impact datasets has shown that EVs have a significantly lower carbon footprint compared to internal combustion engine vehicles, especially when powered by renewable energy.
- Policy Implications: Such insights have driven governments to offer incentives for EV adoption and invest in green energy sources.
Future Trends and the Role of EV Datasets
The future of EV data analysis is promising, with several trends shaping the landscape:
1. Integration with Smart Grids
- As electric vehicles become more common, there’s a growing need to integrate them with smart grids. Datasets will play a crucial role in predicting energy demand and optimizing grid operations.
2. Vehicle-to-Grid (V2G) Technology
- Concept: V2G technology allows EVs to return excess energy to the grid, stabilizing energy supply. Datasets tracking energy flow and consumer behavior will be essential for implementing V2G systems.
3. Impact of Autonomous Vehicles
- Autonomous electric vehicles will generate massive amounts of data. Managing and analyzing this data will be critical for traffic optimization, safety improvements, and environmental assessments.
4. Global Policy Shifts
- Countries are setting ambitious targets to phase out internal combustion engines. Tracking the impact of these policies will require robust datasets on EV sales, emissions, and economic impacts.
FAQs About Electric Vehicle Dataset
Conclusion
Electric Vehicle datasets are a goldmine of information, driving innovation and informed decision-making across industries. From optimizing charging infrastructure to predicting battery life and assessing environmental benefits, these datasets are essential for building a sustainable future. However, challenges like data quality and privacy must be addressed to unlock their full potential. As the EV landscape continues to evolve, so too will the importance and complexity of data analysis.
Whether you’re a data scientist, an automotive expert, or a policymaker, understanding and utilizing EV datasets will be crucial in shaping the future of transportation.